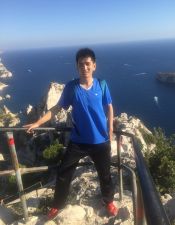
Thesis Project Description:
In this thesis project, we propose to develop deep learning methods to extract instrumental and observational noises from astronomical images and apply these methods to analyze large sky surveys in an automatic manner. Deep learning methods such as style transfer and noise transfer will be studied and developed. For astrophysics, these methods will allow automatic analyses (classification, regression) of cleaned images and noises on sky image simulations. They will be particularly useful for studying the features of millions of galaxies and extracting information about the formation of large scale structures, as well as accurately detecting transient objects (such as supernovae). In the field of computer science, work will be down to develop the deep learning methods for style transfer and noise transfer, aiming at a better understanding of the behavior of neural networks and improvements on their performances. This will naturally lead to extensions in other areas such as photometry and image recognition in underwater environments.
Thesis Supervisors:
Interdisciplinary Research Axis:
Big Data and Imaging
Academic Background:
Tsinghua University (CN)